Machine Learning, Signal Processing and Information Theory
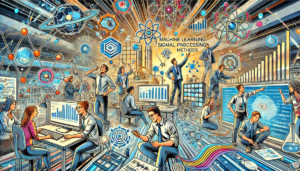
The application of machine learning, signal processing, and information theory methods to communication systems represents a promising avenue for enhancing communication performance. Our research extends beyond the mere application of these methods, encompassing a fundamental understanding and improvement of the methods themselves.